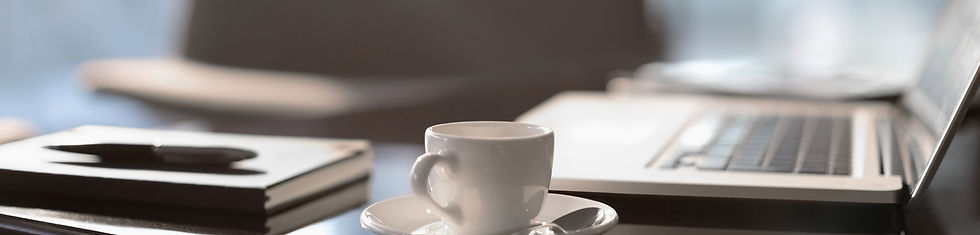
Insights
The Role of Statistics in Data Analysis: A Deep Dive
By
Suma M N
From descriptive statistics to inferential statistics to probability theory, the principles of statistics underpin every aspect of the data analysis process.
Introduction: In the realm of data analysis, statistics stands as the bedrock upon which insights are built, hypotheses are tested, and decisions are made. It serves as the language of uncertainty, enabling analysts to make sense of the chaos within datasets. From identifying trends to making predictions, statistics plays a pivotal role in every step of the data analysis process. In this deep dive, we'll explore the indispensable role of statistics in data analysis, uncovering its significance, key concepts, and practical applications.
Understanding the Basics of Statistics: At its core, statistics is the science of collecting, analyzing, interpreting, and presenting data. It provides a framework for summarizing and understanding the variability and uncertainty inherent in real-world phenomena. To embark on our deep dive, let's first acquaint ourselves with some fundamental concepts of statistics:
Descriptive Statistics: Descriptive statistics involve methods for summarizing and describing the characteristics of a dataset. This includes measures of central tendency (e.g., mean, median, mode) and measures of dispersion (e.g., range, variance, standard deviation). Descriptive statistics provide a snapshot of the data's distribution and help analysts gain insights into its overall structure.
Inferential Statistics: Inferential statistics, on the other hand, involve making inferences or predictions about a population based on a sample of data. This includes hypothesis testing, confidence intervals, and regression analysis. Inferential statistics allow analysts to draw conclusions beyond the observed data and make informed decisions with a certain degree of confidence.
Probability Theory: Probability theory forms the foundation of statistics, providing a framework for quantifying uncertainty and randomness. It allows analysts to model the likelihood of various outcomes and make probabilistic statements about future events. Probability distributions, such as the normal distribution and the binomial distribution, play a crucial role in statistical analysis, providing a mathematical representation of random variables.
Practical Applications of Statistics in Data Analysis: Now that we've established a basic understanding of statistics, let's explore its practical applications in the field of data analysis:
Exploratory Data Analysis (EDA): Exploratory data analysis involves visually exploring and summarizing datasets to uncover patterns, trends, and relationships. Techniques such as histograms, scatter plots, and box plots are used to gain insights into the data's distribution and identify potential outliers or anomalies.
Hypothesis Testing: Hypothesis testing is a statistical method used to determine whether there is enough evidence to support a claim about a population parameter. By formulating null and alternative hypotheses and conducting significance tests, analysts can make informed decisions based on the observed data.
Regression Analysis: Regression analysis is a statistical technique used to model the relationship between one or more independent variables and a dependent variable. It allows analysts to quantify the strength and direction of the relationship and make predictions about future outcomes. Regression models, such as linear regression and logistic regression, are widely used in various fields, including economics, finance, and healthcare.
Predictive Analytics: Predictive analytics involves using statistical models to forecast future events or behaviors based on historical data. Techniques such as time series analysis, machine learning, and data mining are used to identify patterns and make predictions about future trends. Predictive analytics is used in a wide range of applications, from sales forecasting to risk management to healthcare decision-making.
Conclusion: In conclusion, statistics serves as the backbone of data analysis, providing the tools and techniques necessary to extract insights, test hypotheses, and make informed decisions. From descriptive statistics to inferential statistics to probability theory, the principles of statistics underpin every aspect of the data analysis process. By mastering the fundamentals of statistics and applying them judiciously, analysts can unlock the full potential of their data and drive meaningful outcomes. So, whether you're exploring trends in a dataset, testing a hypothesis, or making predictions about the future, remember that statistics is your trusted companion on the journey of data analysis.